
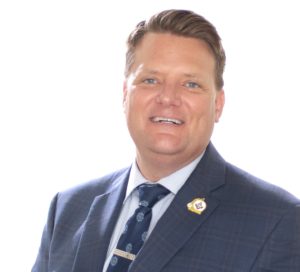
In late 2020, I applied for a technology grant through a machine-learning technology company that builds customized, machine-learning tools based on a facility’s retrospective EHR and rehospitalization data.
My organization, Masonic Pathways, a Life Plan Community in the heart of Michigan, is no stranger to understanding the importance of reducing resident rehospitalization rates.
We currently participate as one of 11 post-acute care providers within the Mid-Michigan Health Performance Network. We are proud to be a member of the performance network, and we do not want to miss out on any opportunity to support our local acute care hospital system and improve patient care.
As a network member, we receive monthly quality scorecard ratings and training on how to decrease overall length of stay and reduce our rehospitalization rates. The network is managed by Signify Health, one of the largest Bundled Payment for Care Improvement Advance conveners in the United States.
As post-acute care organizations grapple with a multitude of strategic challenges, those conveners and acute care hospital systems continue to put the pressure on post-acute care providers to reduce overall rehospitalizations within their communities.
At the same time, the COVID-19 pandemic has plagued our community with low occupancy and a multitude of financial challenges, and, as a result, rehospitalizations just have not risen to the same degree of urgency as other important matters.
To be honest, I understand the importance of managing rehospitalizations. But without an affordable technological solution to assist our team in managing our residents, we continued to “dig into” resident charts in the hope of finding some trends or clues to assist us in improving our rates.
Given my challenges and our desire to strengthen our relationship with not only Mid-Michigan Health Performance Network, but also with Signify Health, I realized our organization needed a technological solution.
Finding the risk
Through the grant program, we gained access to SAIVA Healthcare’s machine learning approach. They build solutions specific to each partnering organization, with the system pulling daily data updates and prospectively running them through the model.
The output is a daily list of residents who are at high risk of rehospitalizations over the next several days. Essentially, the machine-learning technology does the work of pouring through the resident’s chart to find trends and behaviors that predict when a resident is at risk of being rehospitalized.
Now, just because we have machine learning scrubbing our EMR does not mean that we do not have to think. On the contrary, SAIVA reports help us identify early signs of imminent decline and rehospitalization risk, but organizations must still have the human critical thinking skills and clinical acumen to optimize the predictive model.
What we learned through utilizing machine learning is that the charge nurse and care staff MUST document well and provide as many details within the resident chart, so that the machine learning can accurately predict who may rise on the daily ranking report and who may be most at risk for rehospitalization.
The non-tech factor
While predicting imminent decline and reducing rehospitalization risk are the primary focal points of the machine-learning technology, the interdisciplinary team must ask themselves these questions when reviewing their daily ranking reports:
• Do I agree with the SAIVA assessment for this resident? Why or why not?
• If a resident has been on the list previously, is risk going up or down?
• Why might this resident be on the SAIVA list?
• What can I do to reduce the risk to this resident?
• Who can help me reduce the risk to this resident?
I must admit: I am not always a trailblazer for new technology. However, I also must tell you that through machine learning, we can save time and effort and strengthen our overall clinical acumen by analyzing the daily reports. I am pleased to say that, based upon our first couple of months using machine learning, we improved our overall percentage of new resident admission rehospitalizations from 20% to 10.5%. That was in a three-month period.
More importantly, while machine learning is becoming more predominant within healthcare, organizations still need to rely on the human intuition and instincts as well.
Sometimes healthcare professionals are concerned that machine technology might replace them. They need not worry. The only way that we can meaningfully reduce the rehospitalization rate is through close cooperation between machine technology and healthcare professionals.
Mike Logan has a senior living operations management background that spans over 20 years. He is the CEO of Michigan Masonic Home in Alma, Michigan, and a board member of LeadingAge Michigan, PACE Central Michigan and the National PACE Association Finance Committee.